Author: Anurag
11 Data Mining Algorithms

1. Regression & Classification
- Linear
- Multivariate Linear
- Logistic
- Softmax
- Vectorization
- Gradient Calculation
- Stochastic Gradient Descent (SGD)
- Optimizers and Objectives
2. Regularization
- Ridge regression
3. Clustering
k – Means- EM Algorithms
4. Unsupervised Learning
- Autoencoders
- PCA Whitening
- sparse coding
5. Neural Network
- Perceptrons
- Backpropagation
- Restricted Boltzmann Machines
- Learning Vector Quantization
6. Deep Learning
- Stacked Autoencoders
- Convolution Neural Networks (Feature Extraction, Pooling)
- Deep Boltzmann Machines
- Deep Belief Networks
7. Decision Trees
ID3- C4.5
- CART (Classification and regression tree)
- Random Forests
8. Bayesian
Naïve Bayes- Gaussian Naïve Bayes
- Bayesian Networks
- Conditional Random Fields
- Hidden Markov Models
9. Others
- Support Vector Machines
- Evolutionary Methods
- Reinforcement Learning
- Conditional Random Fields
10. Dimensionality Reduction
- PCA
11. Ensemble Methods
- Boosting
- Bagging
- Adaboost
Python Libraries for Data Science
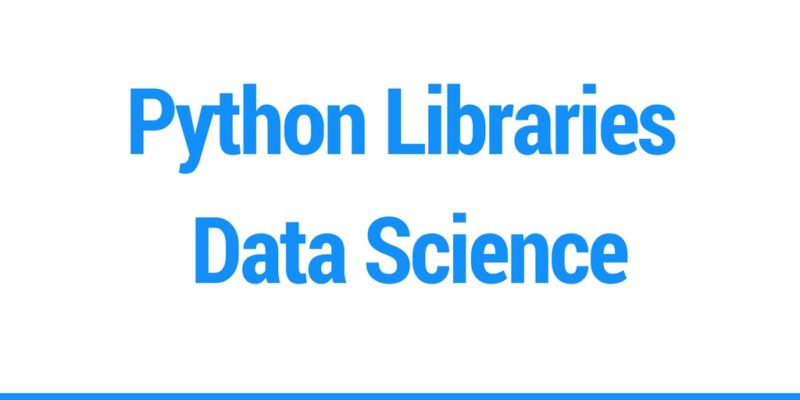
Data Analysis – Machine Learning
Pandas (data preparation)
Pandas help you to carry out your entire data analysis workflow in Python without having to switch to a more domain specific language like R. Practical real world data analysis, reading and writing data, data alignment, reshaping, slicing, fancy indexing, and subsetting, size mutability, merging and joining, Hierarchical axis indexing, Time series-functionality.
See More: Pandas Documentation
Scikit-learn (Machine Learning)
- Simple and efficient tools for implementing Classification, Regression, Clustering, Dimensionality Reduction, Model Selection, Preprocessing.
- Built on NumPy, SciPy, and Matplotlib.
See More: Scikit-learn Documentation
Gensim (Topic Modelling)
Scalable statistical semantics, Analyse plain-text documents for semantic structure and Retrieve semantically similar documents.
See More: Gensim Documentation
NLTK (Natural Language Processing)
Text processing libraries for classification, tokenization, stemming, tagging, parsing, and semantic reasoning, wrappers for industrial-strength NLP libraries. Working with corpora, categorising text, analysing linguistic structure.
See More: NLTK Documentation
Tables
Package for managing hierarchical datasets which are designed to efficiently cope with large amounts of data. It is built on top of the HDF5 library and the NumPy package and features an object-oriented interface which is fast, extremely easy to use tool for interactively save and retrieve large amounts of data.
See More: Tables Documentation
Deep Learning
Deep Learning is a new area of Machine Learning research, which has been introduced with the objective of moving Machine Learning closer to one of its original goals: Artificial Intelligence.
See More: Deep Learning Documentation
Data Visualization
Seaborn
Seaborn is a Python visualisation library based on Matplotlib. It provides a high-level interface for drawing attractive statistical graphics.
See More: Seaborn Documentation
Matplotlib
It is a Python 2D plotting library which produces publication quality figures in a variety of hardcopy formats and interactive environments across platforms. Matplotlib can be used in Python scripts, the Python and IPython shell, the jupyter notebook, web application servers, and four graphical user interface toolkits.
See More: Matplotlib Documentation
Bokeh
Bokeh is a Python interactive visualisation library that targets modern web browsers for presentation. Its goal is to provide elegant, concise construction of novel graphics in the style of D3.js, and to extend this capability with high-performance interactivity over very large or streaming datasets. Bokeh can help anyone who would like to quickly and easily create interactive plots, dashboards, and data applications.
See More: Bokeh Documentation
Sci-py (data quality)
Python library used for scientific computing and technical computing.
SciPy contains modules for optimisation, linear algebra, integration, interpolation, special functions, FFT, signal and image processing, ODE solvers and other tasks common in science and engineering.
SciPy builds on the NumPy array object and is part of the NumPy stack which includes tools like Matplotlib, pandas and SymPy.
See More: Sci-py Documentation
Big Data/Distributed Computing
Hdfs3
hdfs3 is a lightweight Python wrapper for libhdfs3, to interact with the Hadoop File System HDFS.
See More: Hdfs3 Documentation
Luigi
Luigi is a Python package that helps you build complex pipelines of batch jobs. It handles dependency resolution, workflow management, visualisation, handling failures, command line integration, and much more.
See More: Luigi Documentation
Hfpy
It lets you store huge amounts of numerical data, and easily manipulate that data from NumPy. For example, you can slice into multi-terabyte datasets stored on disk, as if they were real NumPy arrays. Thousands of datasets can be stored in a single file, categorised and tagged however you want. H5py uses straightforward NumPy and Python metaphors, like dictionary and NumPy array syntax. For example, you can iterate over data sets in a file, or check out the .shape or .dtype attributes of datasets.
See More: H5py Documentation
Pymongo
PyMongo is a Python distribution containing tools for working with MongoDB, and is the recommended way to work with MongoDB from Python.
See More: PyMongo Documentation
DASK
Dask is a flexible parallel computing library for analytic computing. Dask has two main components Dynamic task scheduling optimised for computation. This is similar to Airflow, Luigi, Celery, or Make, but optimised for interactive computational workloads.“Big Data” collections like parallel arrays, data frames, and lists that extend common interfaces like NumPy, Pandas, or Python iterators to larger-than-memory or distributed environments. These parallel collections run on top of the dynamic task schedulers.
See More: Dask Documentation
Dask.distributed
Dask.distributed is a lightweight library for distributed computing in Python. It extends both the and concurrent.futures
dask
APIs to moderate sized clusters. Distributed
serves to complement the existing PyData analysis stack to meet the following needs Low latency, Peer-to-peer data sharing, Complex Scheduling, Pure Python, Data Locality, Familiar APIs, Easy Setup.
See More: Dask.distributed Documentation
Security
- cryptography
- pyOpenSSL
- passlib
- requests-oauthlib
- ecdsa
- pycrypto
- oauthlib
- oauth2client
- wincertstore
- rsa
So these are some of the Python Libraries for Data Science, data analysis, Machine Learning, Security and Distributed computing.
If you think i miss out Something, let me know in the comments.
Google Data Studio dashboarding and reporting tool

The new Dashboarding and reporting tool Google Data Studio which was launched in 2016 is now available for free for small business earlier it was limited to 5 data reports, now the limit has been lifted from 5 to unlimited.
Data Studio turns your data into informative Dashboards and Reports which you can read, easy to share, and fully customizable. With Dashboarding you can tell great data stories to support better business decisions.
“ To enable more businesses to get full value from Data Studio we are making an important change — we are removing the 5 report limit in Data Studio. You now create and share as many reports as you need — all for free. “
Data Processing Command Line Tools
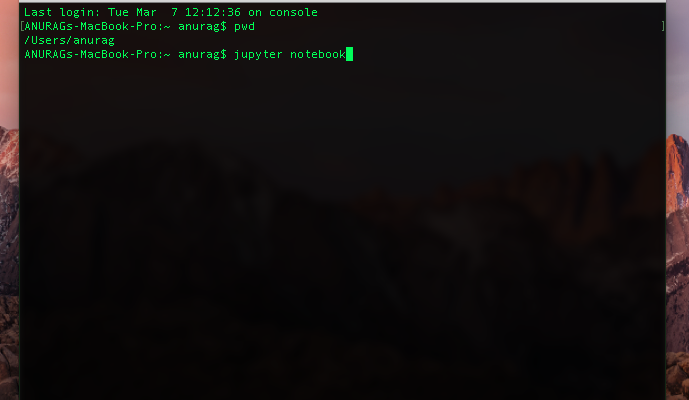
A series of operations on data, to retrieve, transform or classify information, Also the collection and manipulation of items of data to produce meaningful information are known as Data Processing, Some useful Data Processing Command Line tools.
Alternative to Numpy and pandas that solve real-world problems with readable code.
Python library of HTML to IMG wrapper.
Converts an XML input to a JSON output.
Converts a stream of newline separated json data to csv format.
Read data from image – Optical Character Recognition Library.
K Nearest Neighbour & Logistic Regression: Iris Dataset
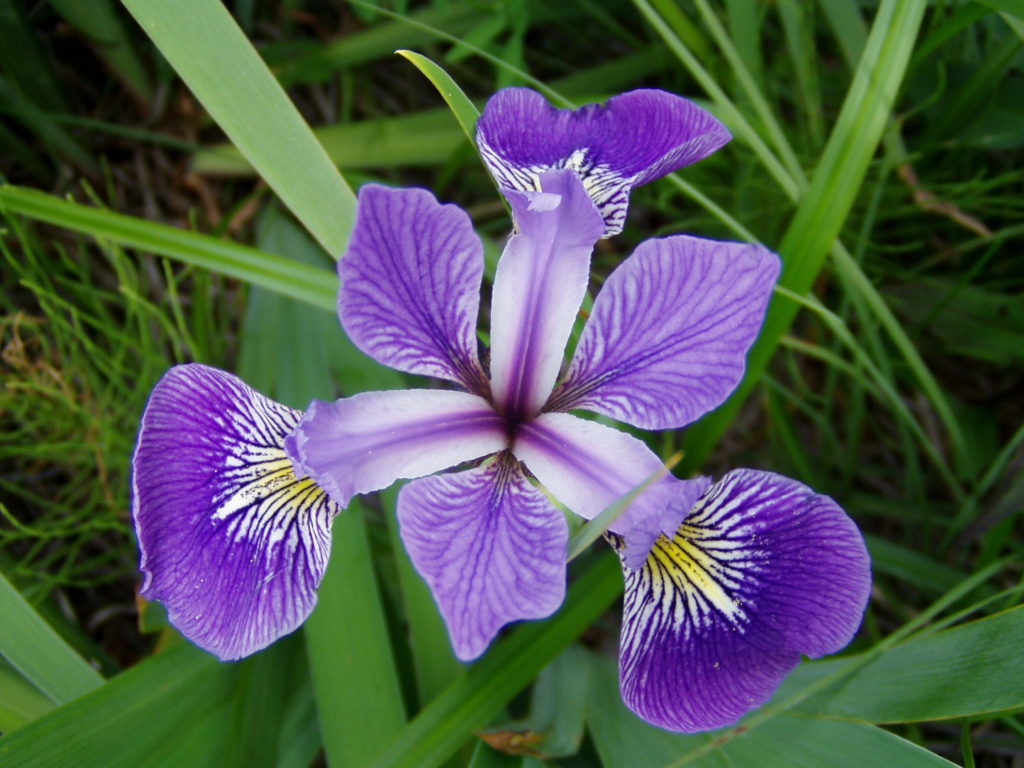
Predicting the Flower Species using K Nearest Neighbour & Logistic Regression on Iris Dataset
Linear Regression: Advertising Dataset

Machine Learning Model of advertising dataset using Linear Regression in Python
CAP Theorem: Big Data
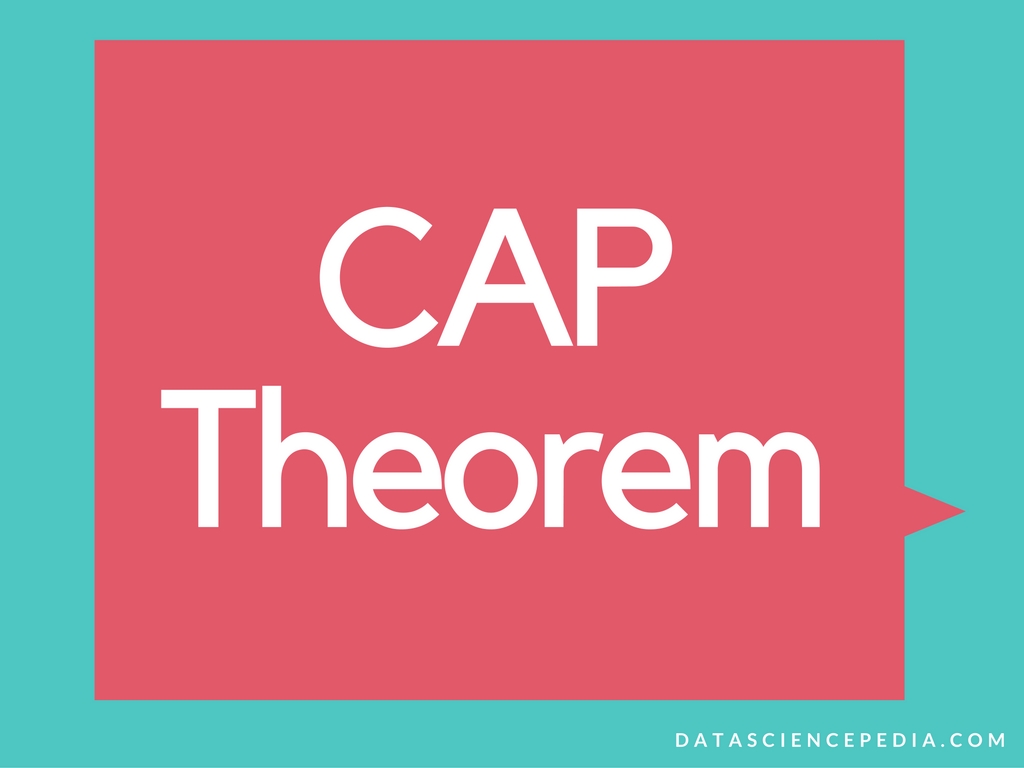
When you’ll start working with NoSQL databases, sooner or later you come across CAP theorem, The theorem published by Eric Brewer in 2000, that describe any distributed system.
In a distributed database system C stands for Consistency, A stands for Availability and P stands for Partition Tolerance.
- Consistency – Every node in the system will have the same view of data.
- Availability – User can read & write from any node.
- Partition Tolerance – Your system will still Operate even if any node or server fails.
So from the above illustration while selecting your NoSQL database you can choose only two characteristics i.e. A-P, A-C, C-P.
A-P Databases: Voldemort, Cassandra, Riak, CouchDB, Dynamo
A-C Databases: PostgreSQL, MySQL
C-P Databases: HBase, MongoDB, Redis, Big Table
NLTK – Natural Language Processing in Python
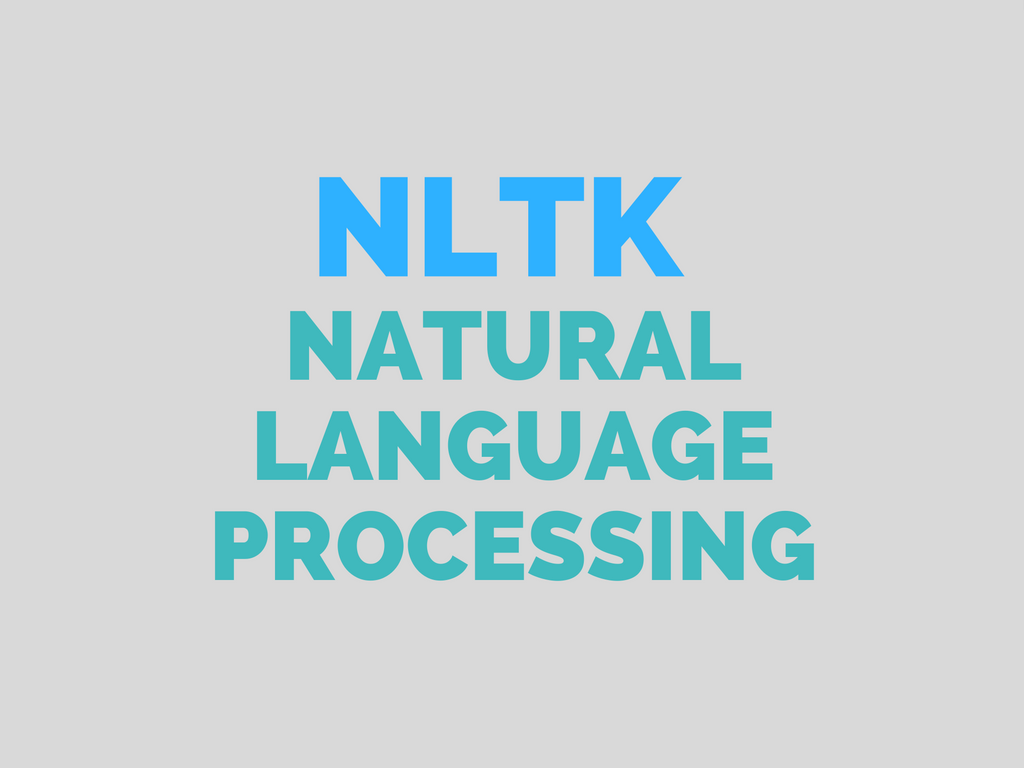
In our day-to-day life we generate a lot of data like tweets, facebook posts, comments, Blog posts, articles which are generally in our natural language and which falls in category of semi-structured and unstructured data, So as when we process natural language data “the unstructured data – plain text” we call it Natural Language Processing.
Natural Language Tool Kit is a library for NLP which deals with natural language such as plain text, words, sentences.
Building blocks of NLTK
- Tokenizers – Separating the text in to words and sentences
word tokenizer – separate by word
sentence tokenizer – separate by sentence
- Corpora – body of text such as any written speech, news article.
- Lexicon – dictionary, meaning of the words. which can be differ in context they are used.
let’s understand how the NLTK works, consider a sample_text such as
So NLTK comes to the rescue and separate the body of text (Corpora) in to sentences & words like